Exploring the Significance of Automated Underwriting Systems
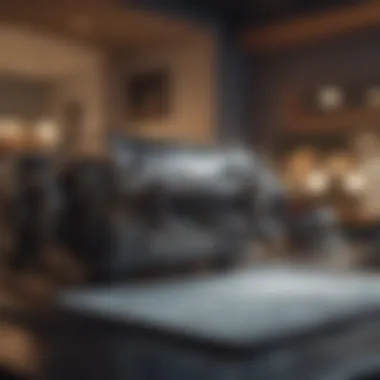
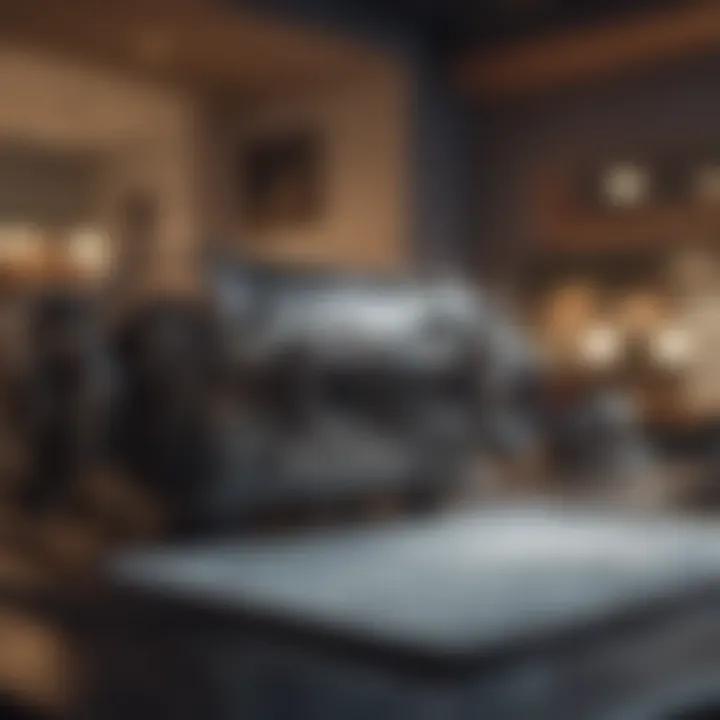
Intro
Automated Underwriting Systems, or AUS, have disrupted traditional lending practices. They work to enhance efficiency in assessing credit risk, providing quicker decisions in lending processes. Understanding the functions and implications of AUS is essential for borrowers and industry professionals alike.
Understanding Credit Scores
Importance of Credit Scores
Credit scores play a critical role in the automated underwriting process. They are numerical representations of an individual's creditworthiness. Lenders heavily rely on these scores to evaluate potential borrowers. A higher score often leads to better loan terms, including lower interest rates.
Factors Affecting Credit Scores
Multiple factors influence credit scores, including:
- Payment history: Timely payments boost your score, while late payments damage it.
- Credit utilization: Using a high percentage of available credit can lower your score.
- Length of credit history: Older credit accounts positively influence your score.
- Types of credit: A mix of installment loans and revolving credit can improve your score.
- Recent inquiries: Multiple hard inquiries within a short period can negatively impact your score.
Tips to Improve Credit Scores
Improving your credit score requires a strategic approach:
- Make payments on time to establish a favorable payment history.
- Keep credit utilization below 30% of your total credit available.
- Avoid opening multiple new credit accounts at once.
- Regularly review your credit report for errors and dispute inaccuracies.
Exploring Automated Underwriting Systems
Mechanics of AUS
Automated Underwriting Systems review borrower information in real-time. They analyze applications, various data points, and integrate advanced algorithms. This technology minimizes the time needed for credit assessment while ensuring it is comprehensive. However, comfort with technology must balance trust in the human element of underwriting decisions.
Benefits of AUS
The primary advantages of AUS are:
- Speed: Faster loan approvals enhance customer experience.
- Consistency: AUS provides standardized evaluations maintaining fairness across applications.
- Efficiency: Reduces operational costs for lenders, facilitating better rates for borrowers.
Challenges In Using AUS
Despite benefits, AUS encounters challenges:
- Over-reliance on data: Technologies can overlook unique borrower situations.
- Security concerns: Safeguarding sensitive data is a continuous process.
- Regulatory compliance: Navigating various regulations can be complex.
The evolution of technology will likely assert more influence over lender decisions, with ongoing updates shaping how risk assessment is conducted.
The Future of Automated Underwriting Systems
The future indicates a more integrated approach in lending technologies. As machine-learning techniques evolve, they will refine the models used in AUS. Lenders who invest in this technology will need to stay updated on best practices and industry standards to maximize efficiency.
Preface to Automated Underwriting Systems
Automated underwriting systems (AUS) represent a pivotal advancement in the lending landscape. Their significance lies in the transformation they offer in the evaluation and decision-making processes related to credit. The efficiency, accuracy, and overall data-driven capabilities that AUS provide make them an essential focus for both financial institutions and consumers. In this digital age, credit processing is evolving, demanding smart and quick solutions. AUS facilitate this transition by allowing for quicker loan approvals and elucidating the underlying criteria involved in risk assessment.
Definition of Automated Underwriting
Automated underwriting refers to the software programs and algorithms used to assess the creditworthiness of potential borrowers. These systems gather and analyze various types of data, allowing lenders to determine the risk associated with granting a loan. Automated underwriting compares both qualitative and quantitative information, rapidly assessing if a loan meets pre-defined guidelines. This process vastly outperforms traditional methods, reducing wait times while maintaining critical evaluation standards that lenders must uphold.
Historical Context
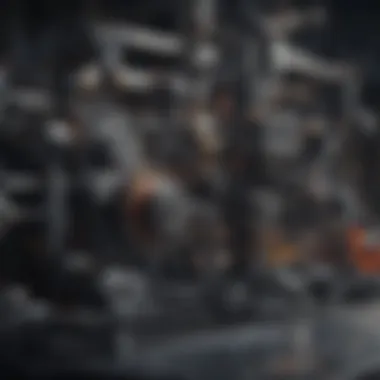
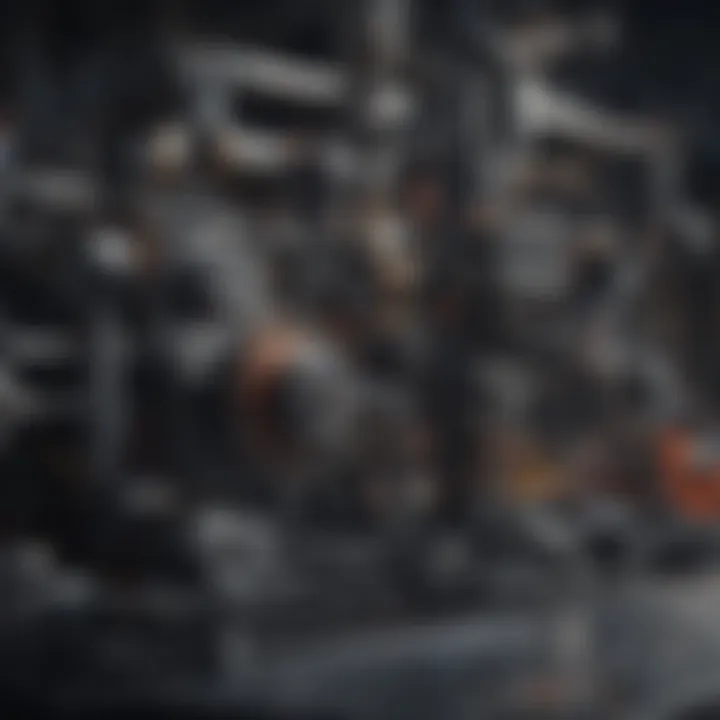
The roots of automated underwriting can be traced back to the increasing integration of technology within financial services. The need for faster loan processing times and improved accuracy began to burgeon in the late 20th century. Traditional underwriting relied heavily on human analysts, leading to potential biases and inconsistencies across different cases.
With the advent of computerized systems in the early 1990s, fundamental shifts hurried into the underwriting process. Commercial interests experienced benefits in efficiencies realized from reducing time spent on each application. Since then, AUS have evolved into sophisticated platforms utilizing machine learning and predictive analytics. These developments bolstered their role amid greater regulatory scrutiny aimed to foster equality in lending practices. This evolution highlights the increasing reliance on technology to navigate consumer demand and market shifts, addressing both financial health and ethical considerations in the borrowing landscape.
Mechanisms of Automated Underwriting Systems
Understanding the mechanisms behind automated underwriting systems (AUS) plays a vital role in realizing their impact on credit evaluations and loan origination. The mechanics include the collection of data, its processing through algorithms, and the use of various risk assessment techniques. Each aspect contributes to enhancing efficiency and ensuring a consistent analysis.
Data Requirements
Data is the cornerstone of automated underwriting. The quality and type of data directly affect the outcomes of the underwriting process. The most common data requirements for AUS include:
- Personal identification information: This often includes the applicant's Social Security number, full name, and contact details.
- Credit history: Credit scores from agencies like FICO or VantageScore provide a snapshot of the borrower’s creditworthiness.
- Income verification documents: Automated systems often require employment records or bank statements to assess financial stability.
- Debt obligations: This can include existing loans, leases, and credit card obligations to determine debt-to-income ratios.
The availability and accuracy of this information are crucial. Inaccurate data can discriminatorily affect one's credit rating or loan eligibility. Applications relying on outdated or erroneous data have a lesser probability of generating fair outcomes. Therefore, it is critical for lenders to perform due diligence when selecting data providers and ensuring data is current and comprehensive.
Algorithmic Processes
Once the necessary data is gathered, algorithmic processes take over. These algorithms are designed to analyze input data rapidly and reliably. Various components contribute to the effectiveness of these algorithms:
- Decision Rules: These are systematic approaches that align data points with risk factors defined either by historical performance or regulatory guidelines.
- Computational Models: Machine learning models can adapt based on experience, improving the decision-making process over time. They predict outcomes using vast datasets to uncover trends that manual methods might miss.
- Scoring Mechanisms: Scoring is critical in determining approval or rejection of loan applications. Changes within scoring algorithms often evolve based on feedback and new data trends, presenting both opportunities and challenges for stakeholders.
The key to an effective algorithm is not only accuracy but also transparency. Stakeholders need to understand how adjustments are made. If borrowers or regulators cannot comprehend the models utilized, distrust may ensue.
Risk Assessment Techniques
Risk assessment is at the heart of any underwriting process. In the context of AUS, several risk assessment techniques are commonly employed:
- Model Risk Evaluation: Evaluating models to avoid overfitting through validation datasets will assure accuracy in distinguishing between credit-worthy and non-credit-worthy profiles.
- Scenario Analysis: This technique examines various potential borrower situations, helping lenders identify varying risk levels among applicants and anticipate stress factors affecting borrower repayment ability.
- Predictive Analytics: This examines patterns emanating from historical data, influencing future lending decisions. Insights from predictive analytics enable improvements in borrower evaluation and help anticipate downturns in borrower risk areas.
Effective risk assessment within AUS not only reduces credit loss but also enhances strategic decision making.
Overall, each of these mechanisms, ranging from stringent data requirements, advanced algorithmic processes, to comprehensive risk assessment techniques, emphasizes the complexity behind an automated underwriting system. Understanding this complexity permits stakeholders to make informed decisions, which is crucial for both lending institutions and borrowers seeking to optimize their loan requests.
Benefits of Automated Underwriting Systems
Automated underwriting systems (AUS) have transformed the lending landscape organized around more objective measures and operational speed. This segment offers an insightful look at the core benefits that AUS streamlines into modern processes. Each benefit possesses distinct characteristics that can enhance risk assessment and decision-making capabilities. Borrowers, lenders, and financial institutions increasingly recognize the significance of these implementations in improving many facets of the underwriting process.
Increased Efficiency
The primary advantage of AUS is the tremendous efficiency it brings to underwriting. Traditional underwriting relies heavily on underwriters who assess loan applications, which often creates potential delays. Manual processing may increment the underwriting time and hinder competition among lenders. Review of documents involving a manual process may require comprehensive back-and-forth communication with borrowers. On the contrary, automating these functions reduces the overall processing timeline significantly.
An efficient AUS can usually complete underwriting decisions in minutes. The system analyzes applicant data, ensuring quick evaluations. Speed advantages lead to operations functioning more smoothly. Faster underwriting directly benefits borrowers who need a timely decision regarding loans. Loan commit deadlines become shorter and more manageable, enriching the overall consumer experience.
Enhanced Accuracy
Accuracy serves as an essential prerequisite in ensuring that loans are underwritten correctly. Outcome misjudgments often lead to severe implications for lenders and borrowers alike. Automated underwriting systems come with advanced algorithms that allow for data analysis with high precision. By doing so, they minimize the risk of human error.
AUS objectively process data, factoring in considerations that might be overlooked or miscalculated by a human underwriter. Inconsistencies in applications may become visible through the system. This trait allows creditors to enforce truthful representations from borrowers without placing subjective judgments. Enhanced accuracy guarantees consistency, contributing to reduced risk to financial institutions.
“The overarching goal of automated underwriting is to provide clarity and certainty in the risk involved in lending, assuring inquiries are pitch-perfect.”
Cost Reduction
Automated underwriting systems also present a compelling case for cost reduction. Manual processes require human resource allocations, operational overhead, and continual training for underwriters. AUS decreases the dependency on in-house staff for underwriting, allowing lenders to allocate resources more effectively.
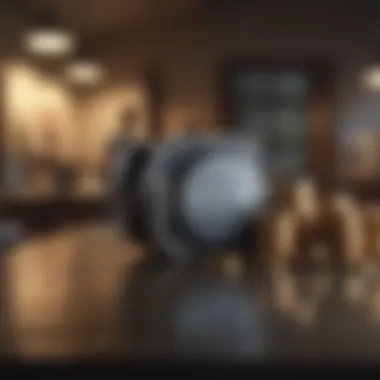
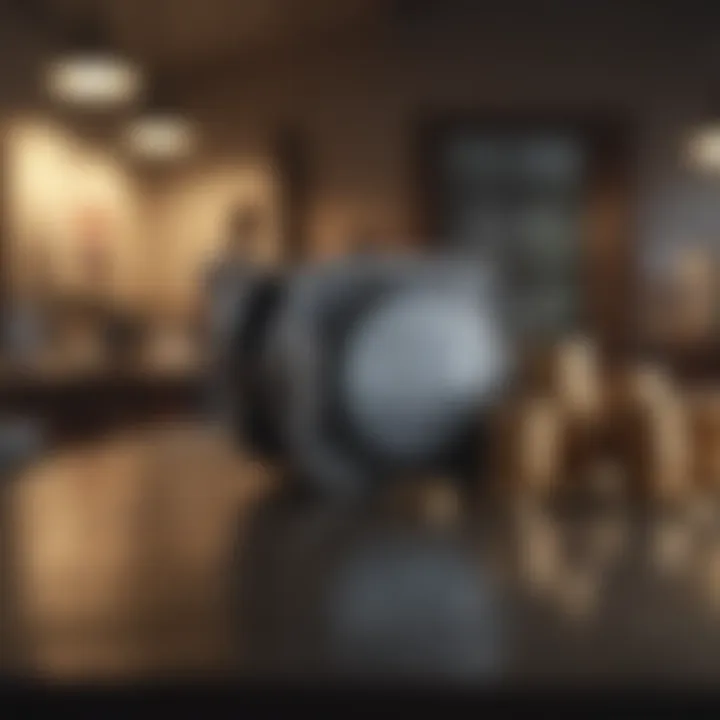
Operational expenses diminish rapidly as the necessity for various administrative efforts declines. Lenders can divert beyond savings gained from salaries to strategic investments in improved technology. Additionally, errors made by human processes invited additional costs and resource allocation to rectify. Notably, with AUS efficiently targeting anomalies and undercorrecting misconceptions during underwriting processes, financial institutions can anticipate improved profit margins subsequent to investment in such systems.
Challenges Associated with Automated Underwriting Systems
Automated underwriting systems (AUS) present numerous advantages. However, navigating their challenges is crucial for users looking to maximize their benefits. Recognizing these issues helps inform decisions in the lending process. Understanding the challenges can lead to better strategies for utilizing automated underwriting solutions. Significant concerns include data privacy, system limitations, and issues involving bias and fairness.
Data Privacy Concerns
As more institutions adopt automated underwriting systems, the volume of sensitive information processed expands. This can lead to increased risk of data breaches and unauthorized access. An important matter is protecting personal financial data. Users must ensure that data processed by AUS is stored securely and handled with care.
Regulations related to data privacy, such as the General Data Protection Regulation (GDPR), are critical in shaping AUS. Failure to comply can result in penalties that affect lenders significantly. Leverage secure systems and encryption can maintain the integrity of borrower information. Addressing these concerns not only builds trust among potential customers but also enhances the reputation of financial institutions.
System Limitations
Though automated underwriting systems offer speed, they are limited by the data and algorithms they utilize. These systems can only assess risk based on available inputs. Inaccurate data can produce flawed outcomes, resulting in poor loan decisions. Financial institutions should review and update data frequently to ensure accuracy.
Moreover, automated systems can misinterpret complex financial profiles. This can jeopardize approval chances for well-qualified borrowers due to oversimplified assessments. It’s important that lenders balance AUS with human judgement for more nuanced evaluations. Recognizing these limitations aids lenders in crafting a more reliable lending framework that optimally deploys AUS.
Bias and Fairness Issues
Bias and fairness in automated underwriting systems require significant attention. Since algorithms are trained using historical data, they may inadvertently perpetuate existing inequalities. A system relying heavily on past behaviors can unfairly disadvantage certain demographics. The quality of credit evaluations becomes flawed if biases in input data are not identified and adequately addressed.
Fairness can be further hindered if systems are not constantly reassessed. Real-world outcomes achieved through AUS should not resemble trends that upheld unjust practices. Systems must proactively combat bias by using inclusive data sources and measurable fairness criteria. It is essential to ensure that loan decisions are based on comprehensive understanding of applicants, rather than automated processes alone.
Addressing challenges in automated underwriting systems is crucial. This ensures that they operate equitably and effectively, shielding institutions from regulatory penalties and preservation of trust.
Comparative Analysis of Traditional vs.
Automated Underwriting
The comparative analysis of Traditional and Automated Underwriting is crucial. Understanding how these two methodologies coexist offers valuable insights into the evolving landscape of lending. Traditional underwriting relies heavily on human discretion and a subjective assessment of a borrower's risk. While effective, this method often faces longer turnaround times and can introduce inconsistencies.
In contrast, Automated Underwriting Systems utilize algorithms and data analytics to evaluate borrower applications. By employing large sets of data, these systems can produce decisions at a much faster pace. This shift is not only transforming processes but also affecting the capacity of lending institutions to cater to diverse borrower needs.
Methodological Differences
In the realm of underwriting, methodologies vary significantly between traditional and automated systems. Traditional methods depend on manual data entry and personal judgment, leading to challenges in terms of bias and error. Underwriters typically review a borrower’s credit history, income verification, and other relevant documents.
Automated Underwriting employs a structured process that removes much of the ambiguity inherent in traditional methods. \n- Data Analysis: These systems analyze various data points. They also employ alternative data sources to provide a more well-rounded assessment into a borrower's risk profile.
- Algorithmic Models: An algorithm-based approach allows for rapid decision-making. These models ensure consistency and accuracy, although they still face scrutiny regarding fairness.
The procedural shifts highlight a significant advancement in how underwriting operates. As technology innovations continue, the approach to handling borrower data will likely become even more sophisticated. This modernization drives demand for speed and efficiency in lending practices, making the data-centric approach indispensable.
Outcomes and Decision-Making
The outcomes derived from each methodology can affect both lenders and borrowers immensely. Traditional underwriting’s reliance on human judgment can lead to subjective conclusions. This inconsistency sometimes affects particular demographics unfairly.
Automated Underwriting Systems provide a noticeable contrast. They generate results based on quantitative assessments. These outcomes often lead to:
- Faster Approval Times: Borrowers can receive decisions in nearly real-time, allowing them to proceed without prolonged waiting periods.
- Improved Accuracy: The empirical nature of automated systems means that statistical trends from large datasets are utilized. This can result in a more equitable lending experience.
- Higher Acceptance Rates: With alternative data usage, more individuals may qualify for credit who would have otherwise been marginalized by traditional scores.
Overall, comparing these two systems illustrates the balance lenders must strike between efficiency and fairness while providing appropriate credit avenues for varied borrowers. Both methodologies serve unique purposes within the lending ecosystem, but the move towards automated systems marks an essential advance in financial technology. Its implications will likely develop alongside borrowing demands and expectations.
The Role of Artificial Intelligence in Automated Underwriting
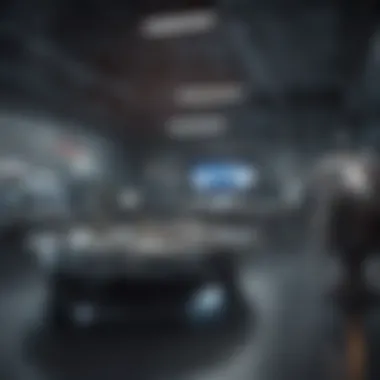
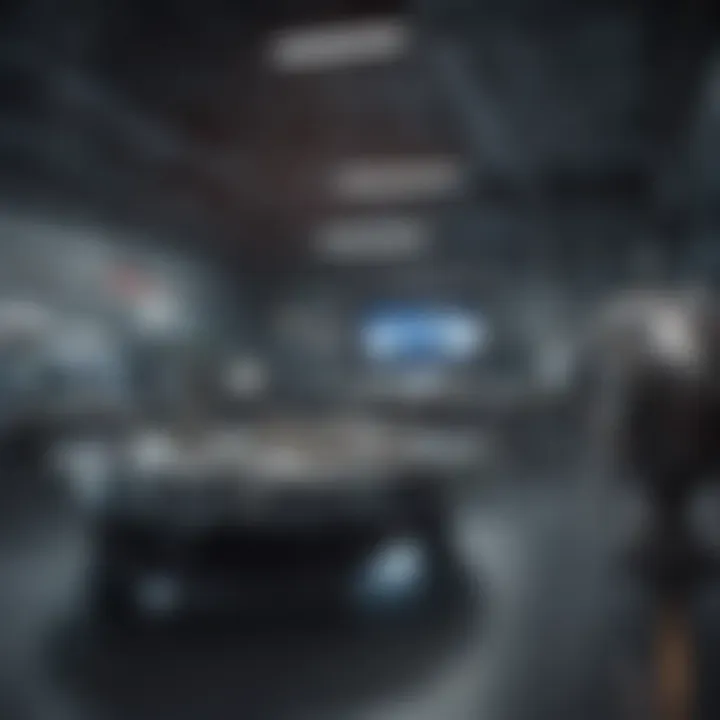
The integration of Artificial Intelligence (AI) within Automated Underwriting Systems (AUS) marks a significant transformation in the lending space. Understanding this integration is critical, as it shapes decision-making processes and influences borrower outcomes. AI contributes to more precise risk analyses, facilitating improved efficiency and operational productivity.
Machine Learning Techniques
Machine Learning (ML) is a core subset of AI that focuses on the development of algorithms capable of recognizing patterns in vast datasets. In the context of automated underwriting, machine learning employs these patterns to evaluate creditworthiness accurately.
- Some key aspects of machine learning techniques in AUS include:
- Data Processing: A vast amount of historical data is employed to train algorithms. This helps the systems learn borrower behaviors and financial backgrounds, allowing for more tailored risk assessments.
- Continuous Improvement: Over time, these algorithms refine their decision-making abilities through ongoing exposure to new data. This means the system can adapt to shifting market conditions and evolving borrower profile trends.
- Enhanced Predictive Capabilities: Machine learning improves the predictive capability of underwriting systems by identifying complex variables and their interactions that might not be apparent through traditional analysis.
By effectively using machine learning techniques, lenders can derive sharper insights that support confident credit decisions while reducing the potential for human error.
Predictive Analytics Applications
Predictive analytics involves using statistical techniques, machine learning, and data mining to identify future outcomes based on historical data. Within automated underwriting, predictive analytics plays a crucial role in enhancing decision accuracy.
- Important applications of predictive analytics in this context include:
- Risk Scoring Models: By assessing various data points, predictive analytics generates risk scores that indicate the likelihood of default. These scores inform lenders' strategies for risk management.
- Customer Behavior Predictions: Predictive analysis allows lenders to understand and anticipate borrower behavior, optimizing marketing efforts based on the likelihood of loan acceptance.
- Fraud Detection: Identifying anomalous patterns through predictive algorithms helps spot fraudulent applications. This directly contributes to safeguarding lender interests and protecting against potential losses.
In making decisions, lenders can leverage these predictive insights to ensure smarter, informed underwriting practices.
The Future of Automated Underwriting Systems
The landscape of automated underwriting systems (AUS) is constantly evolving due to technological developments and regulatory changes. This future trajectory holds significant implications for financial institutions, lenders, and borrowers alike. Understanding the future of AUS will help users harness its potential and navigate challenges that could arise. Embracing innovative solutions ensures better risk assessment, accuracy in credit decisions, and ultimately strengthens borrower-lender relationships in an increasingly competitive market.
Trends and Innovations
The coming years are poised to witness several key trends within automated underwriting systems. As technologies continue to advance, several innovations are likely to become mainstream.
- Increased Use of Artificial Intelligence: The integration of AI capabilities continues to expand. Lenders will utilize complex algorithms and machine learning to analyze vast data sets efficiently. This can refine the credit scoring process and provide more personalized offers to potential borrowers.
- Data Diversification: There is a push to incorporate non-traditional data sources into the underwriting processes. Alternative data, such as payment histories from services like utilities or telecommunication bills, allow AU systems to evaluate borrowers who may lack conventional credit history effectively.
- Real-Time Processing: Quick decisions are vital in a fast-paced financial environment. Future AUS will capitalize on real-time data analytics, enabling lenders to assess the information instantly and respond to applications faster than ever before.
In summary, trends focus on personalization, speed, and broader evaluations, creating a user-friendly underwriting process that adapts to borrower needs.
Regulatory Influences
The regulation landscape surrounding automated underwriting systems is complex and multifaceted. Regulatory bodies will likely shape the different components, governing factors from data protection to algorithms' bias.
- Consumer Protection Regulations: As the underwriting process becomes increasingly automated, ensuring that borrowers' rights are protected will be pivotal. Regulatory measures will be necessary to establish frameworks for transparent decision-making.
- Data Privacy Laws: The collection and utilization of vast amounts of data raise privacy concerns. Upcoming regulations will likely emphasize the importance of consumers’ consent for data usage and proper handling of their sensitive information.
- Bias Mitigation: Addressing biases inherent in algorithms will be crucial to compliance. Regulators may implement requirements to regularly audit and assess AUS for fairness, reducing discriminatory practices while fostering trust in the credit evaluation process.
As automated processes dominate credit decisions, evolving regulations strive to maintain ethical standards that protect both lenders and borrowers.
By understanding the potential challenges nascent regulations face, industry stakeholders prepare to adapt willingly. Fostering a collaborative environment among financial institutions, regulators, and consumers can lead to innovative solutions that positively affect the underwriting landscape.
The End
The conclusion of this article reflects on the essential elements of automated underwriting systems and their transformation of the lending landscape. Understanding the intricacies of AUS is critical for various stakeholders, including lenders, borrowers, and regulators. The significance of AUS lies not just in enhanced efficiency but also in their ability to mitigate credit risk and foster better decision-making.
Summary of Insights
In summary, this article has elaborated on several key aspects of automated underwriting systems. The mechanisms behind these systems include data requirements and algorithmic processes that ensure robust risk assessment techniques. We have also highlighted the numerous benefits that these systems offer, including increased efficiency, improved accuracy in decision-making, and considerable cost reductions.
Furthermore, multiple challenges linked to AUS, such as data privacy issues and potential biases, were examined. An insightful comparison with traditional underwriting methods allowed us to see the limitations as well as the advantages of automation. The role of artificial intelligence was explored, illustrating how machine learning and predictive analytics are paving the future for AUS.
Finally, significant trends and innovations emerging in this field were discussed. Regulatory influences shape the evolution of AUS, demanding ongoing considerations to maintain equitable access to credit.
Final Thoughts
The landscape of credit assessment is evolving. Automated underwriting systems stand as a fundamental advancement in making credit more accessible and efficient. Stakeholders, especially individuals looking to enhance their credit scores, should be aware of these systems' benefits and challenges. By being knowledgeable about how AUS functions, borrowers can better position themselves in the credit market.
It is important to monitor upcoming trends and regulatory changes, as they will continue to impact how lending operates. As people navigate their financial journeys, being informed about automated underwriting systems will empower them to make wise credit decisions. Keeping abreast of future innovations in technology could potentially yield more opportunities for better credit health and financial stability.
Knowledge about AUS has significant value for individuals aiming to improve their creditworthiness and navigate the complexities of borrowing effectively.